Scaling paid acquisition is getting tougher. Costs are rising, tracking is harder, and the old playbooks don’t work like they used to. Marketers need new ways to find and grow high-value users without wasting budgets.
That’s exactly what Peleg Israeli (CRO, Voyantis) and Andrey Shakhtin (CEO, FunnelFox) discussed in the recent webinar.
This article covers the main ideas from that conversation: what’s changed in user acquisition, how predictive AI can help, and what steps marketers can take to drive better results.
You can also watch the full webinar recording.
Biggest challenges in paid UA today
Today’s user acquisition landscape is tougher than ever. Several forces are converging, making it harder to acquire, measure, and optimize high-value users.
UA is more expensive and harder to measure
Advertising costs continue to rise across major platforms. At the same time, measuring user behavior and campaign performance has become increasingly complicated.
The app market is crowded
Competition is fierce. Standing out among thousands of apps demands sharper strategies, better creatives, and smarter optimization.
Privacy changes limit high-value user tracking
Regulations like GDPR and Apple’s AppTrackingTransparency (ATT) framework have fundamentally changed how marketers track users. Data is scarcer, more aggregated, and delayed.
IDFA is mostly gone, and SKAdNetwork is incomplete
The loss of device identifiers (IDFA) means less precise targeting and attribution. While SKAdNetwork offers an alternative, it delivers delayed and aggregated data — far from real-time insights.
Platforms are shifting to AI and automation
Ad platforms like Meta and Google are leaning heavily into AI-driven automation. Manual controls over targeting, bidding, and optimization are shrinking, giving marketers fewer levers to directly influence outcomes.
This shift toward automation has clear benefits, but it also comes with a tradeoff. Traditional bidding strategies are struggling to keep up with the new reality.
The limits of traditional bidding
Traditional bidding models are losing effectiveness in today’s environment. Historically, marketers focused on driving as much volume as possible — clicks, installs, impressions. But volume alone no longer guarantees business outcomes.
A download doesn’t ensure long-term revenue. Users need to be measured not just by the first action they take, but by the value they generate over time.
Legacy strategies like target CPA (tCPA) bidding are becoming outdated for long-term growth. While they still have a place for scaling campaigns quickly, they often miss the bigger picture: identifying and prioritizing high-value users from Day 1.
That’s where Value-Based Optimization (VBO) comes in. By feeding richer, predictive signals to platforms like Meta, Google, and TikTok, marketers can guide the algorithms to find users who are most likely to generate meaningful revenue — not just complete low-value actions.
Today, some marketers are adopting hybrid strategies:
- Using tCPA to drive volume and scale,
- Combining it with VBO or VO models to maximize long-term value and profitability.
The shift is clear: campaigns optimized for value consistently outperform those optimized for volume alone.
New strategies to master creative and signal optimization
As AI takes over targeting, bidding, and optimization, marketers are left with fewer levers to pull. But not everything is out of their hands. There are still two areas where strategic decisions have a major impact:
Creative strategy
Creative assets — not targeting settings — determine user quality and acquisition costs. A structured testing process is critical:
- Generate multiple variations rapidly (with or without AI tools)
- Test systematically against performance metrics
- Scale winning creatives quickly across channels
Signal optimization strategy
Predictive, value-based signals significantly improve platform learning and acquisition efficiency. Core practices:
- Send predicted user value early within the conversion window
- Refresh signals as user behavior evolves
- Build stable feedback loops to reinforce model accuracy
Mastering both pillars leads to faster learning, lower CAC, higher LTV, and sustainable ROAS gains.
What is predictive AI
Predictive AI models forecast a user’s future value based on early actions, demographics, and engagement signals.
Instead of waiting weeks or months to measure real LTV, marketers can estimate it within the first hours or days. These predictions are then fed back to platforms like Meta, Google, and TikTok to guide optimization from the start.
Unlike traditional event-based optimization (e.g., installs, purchases), predictive AI focuses on future behavior:
- Will this user become a paying customer?
- How much will they spend over 30, 60, or 90 days?
- How likely are they to stay engaged or churn?
Feeding predicted user value early allows platforms to find more high-value users faster — improving acquisition efficiency and long-term profitability.
Predictive AI goes beyond LTV prediction
Predictive AI is often associated with LTV modeling, but its applications are broader. Depending on the business model and goals, marketers can predict various user behaviors that correlate with long-term value.
Common predictive targets include:
- Revenue over a defined time horizon (e.g., expected spend over 30, 60, or 90 days)
- Subscription conversion likelihood (e.g., probability of a freemium user upgrading to paid within two weeks)
- Engagement milestones (e.g., number of sessions, completed onboarding steps, or feature usage)
- Retention probability (e.g., likelihood of staying active after 7 or 30 days)
- Cross-sell or upsell potential (e.g., users likely to purchase additional products or services)
Marketers define their Most Valuable User (MVU) profile based on these events — and predictive models forecast each user’s probability to achieve them.
This flexibility allows companies across different verticals — from fintech to health & fitness apps — to optimize for what truly matters to their business, not just immediate transactions.
Which companies benefit most from predictive AI
Predictive AI is most effective for businesses where user value is realized over time, not at the first transaction.
Key verticals that see the strongest gains:
- Fintech and financial services. Long funnels, delayed revenue, and high-value customer journeys make predictive optimization critical.
- Subscription-based products. Whether SaaS, media, or fitness apps, predicting subscription upgrades and long-term retention unlocks major ROAS improvements.
- Product-led growth (PLG) companies. Freemium models with low initial monetization require early identification of users likely to convert to paid plans.
- Lead generation businesses. Where user actions (like a signup) only indirectly lead to monetizable outcomes weeks or months later.
- E-commerce with repeat purchase behavior. Predicting customer lifetime value beyond the first sale supports smarter scaling decisions.
Companies in these categories often achieve 20–50% improvements in acquisition efficiency by implementing predictive AI strategies correctly.
How to send predictive signals effectively
Accurate predictions are just the start. To drive real performance gains, marketers must deliver predictive signals in a way that platforms can understand and act on.
Key factors to manage:
1. Timing matters
Signals must be sent early — ideally within the 7-day conversion window — to influence platform optimization. Delayed signals lose impact.
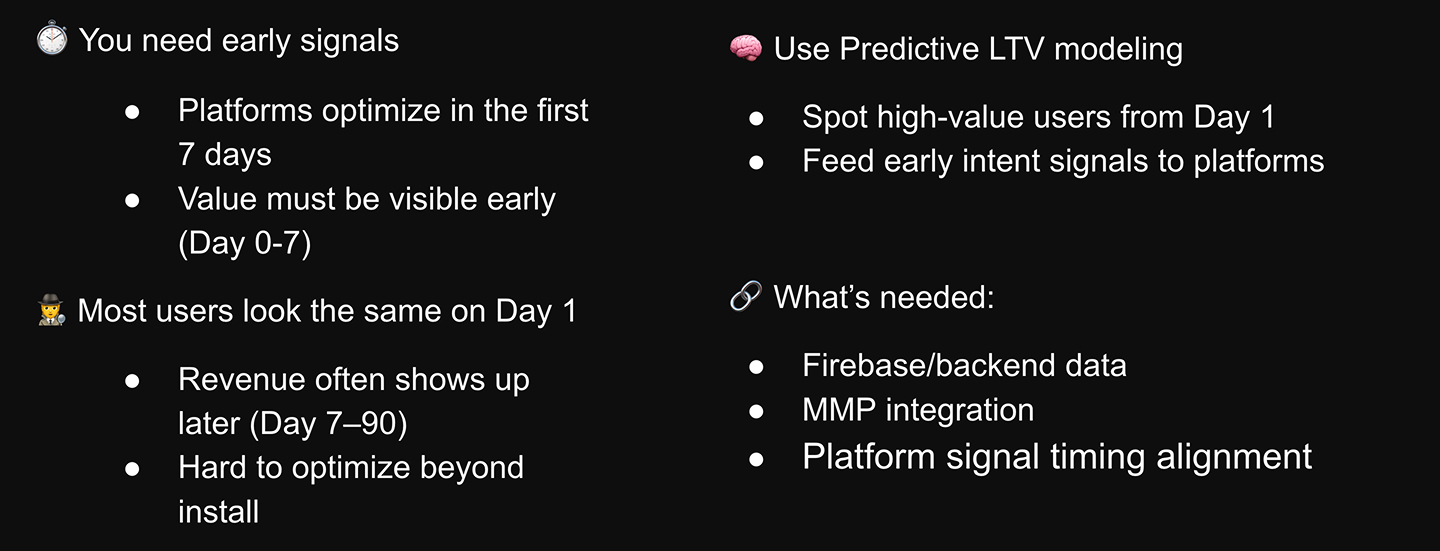
2. Balance accuracy and speed
Waiting longer improves prediction accuracy but reduces optimization influence. Early predictions may be less precise but are more actionable.
3. Incremental signal updates
Instead of sending a full predicted value immediately, marketers can send partial values and refine them as user behavior unfolds.
4. Alignment with platform models
Signals should match the optimization frameworks of each platform (e.g., tROAS for Google, VO for Meta) to maximize learning efficiency.
5. Training platforms over time
Predictive signals require patience. It takes weeks of consistent signal delivery for platforms to adjust their models and improve performance.
Without careful attention to timing, value structure, and learning cycles, even the best predictive models will underperform.
Real-world example: MoneyLion case study
MoneyLion, a leading U.S. fintech company, implemented predictive AI to optimize their paid acquisition.
Challenge
Standard event-based optimization was limiting campaign performance, as early user actions didn’t fully predict long-term value.
Solution
MoneyLion used predictive models to estimate future user value early and fed these signals into Google’s tROAS optimization system.
Results
- Expected ROAS improvement: +10%
- Actual ROAS improvement after full platform learning: +30%
This case shows how predictive AI can unlock major growth — with patience and the right setup.
Common mistakes when implementing predictive AI
While predictive AI can deliver major gains, success depends on careful execution. Some of the most frequent mistakes include:
- Expecting immediate results. Predictive signals need time to work. It usually takes 4–8 weeks of consistent delivery before results improve.
- Sending raw prediction values too early. Early predictions are often less accurate. Sending full-value signals immediately can confuse platform models instead of improving them.
- Failing to manage signal timing. Missing the 7-day conversion window reduces signal effectiveness dramatically.
- Underestimating platform learning processes. Platforms like Meta and Google need gradual, consistent training on new predictive signals to optimize correctly.
- Not aligning signals with platform optimization frameworks. Predictive outputs must match how platforms optimize (e.g., scaled to tROAS or VO models), otherwise performance gains are limited.
Predictive AI is powerful, but it demands precise setup, patience, and close integration with each ad platform’s mechanics to deliver sustainable growth.
What’s next for user acquisition and predictive AI
User acquisition is shifting from growth-at-all-costs to sustainable, profitable scaling.
In this new environment, predictive AI is moving from an experimental tactic to a core strategic advantage. Companies that build strong predictive frameworks — combining early signal delivery, creative excellence, and platform-specific optimization — are pulling ahead.
Over the next 1–2 years, we can expect:
- Wider adoption of predictive optimization across industries
- Increased focus on profitability metrics (not just volume)
- Closer collaboration between marketing, data science, and product teams
- Growing reliance on early engagement signals to drive platform learning
Marketers who master predictive approaches today will be best positioned to navigate tightening budgets, rising competition, and evolving platform dynamics.